In the ever-evolving landscape of search engine optimization (SEO), crafting a strategy that cuts through the noise and delivers content precisely where and when it’s needed is crucial. Enter ByRelevanceFilter – a nuanced approach to SEO that prioritizes relevance and context in content discovery. This comprehensive guide will explore how integrating ByRelevanceFilter into your SEO strategy can enhance visibility, drive engagement, and elevate your online presence.
Different Techniques to Filter Information for Relevance
The transition from data overload to narrowing information by relevance has been a significant shift. A myriad of platforms focus on filtering information to present the most relevant content to their users. But which of these platforms will prevail? Let’s explore the diverse methodologies these platforms use in their quest for content relevance.
These methods are usually the focus of academic research papers, but here we provide a general overview for everyone to understand:
- Algorithmic Filtering;
- Social Graph-based Filtering;
- Human Filtering;
- Crowdsourced Filtering;
- Shared Sources Filtering (Meta);
- Influence Filtering;
- Social Search;
- Location Filtering.
Understanding Algorithmic Filtering
Algorithmic filtering relies on understanding your likes and preferences to predict and present content that suits your interest. The most notable application of this methodology is in web search, particularly with Google’s search algorithm, which sifts through billions of web pages to present you with the most relevant search results.
Google also employs this form of filtering in its “Sort by Magic” feature in Google Reader.
- Advantages: It can provide highly relevant results when searching for information;
- Drawbacks: It lacks spontaneity and offers little to no personalization as search engines are often unaware of the user’s demographic information.
The Power of Social Graph Filtering
The social graph filtering operates on the principle – if it’s liked by your friends, you’ll probably like it too. Various platforms, including Facebook and Google Buzz, employ this approach to recommend content based on your connections’ interactions.
- Advantages: This approach offers high levels of spontaneity and personalization, and it helps users feel connected;
- Drawbacks: Its effectiveness highly depends on the quality of the social graph, which is often not optimized for relevance.
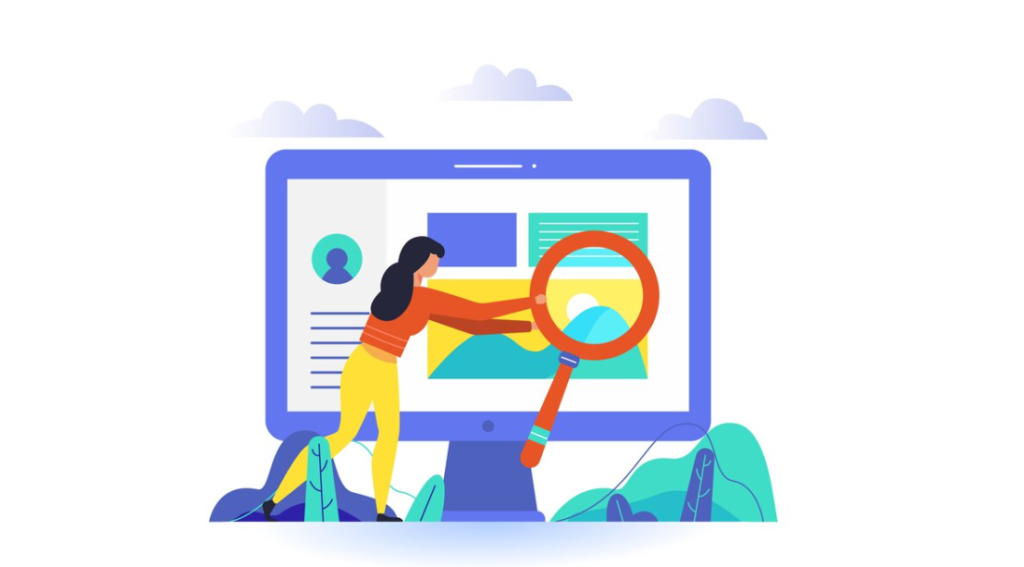
The Role of Human Filtering in Content Relevance
Human filtering places trust in certain individuals whom users find reliable as news sources.
- Advantages: It offers high spontaneity and helps users quickly become part of an influencer’s social circle;
- Drawbacks: It can be unreliable and heavily subject to a person’s preferences and agenda.
The Impact of Crowdsourced Filtering
Platforms like TweetMeme, OneRiot, Digg, and many other social bookmarking services utilize crowdsourced filtering to highlight the most popular content.
- Advantages: It helps users stay updated with the most popular and trending information;
- Drawbacks: It lacks personalization as the popular content doesn’t necessarily equate to relevant content.
Shared Sources Filtering (Meta) Explained
This approach suggests that if you read from sources similar to someone else, you’ll probably like their other sources too. Platforms like Facebook and Google Reader use this approach to recommend new pages or feeds you might be interested in.
- Advantages: Great for uncovering new sources on social networks;
- Drawbacks: It filters sources, not actual news items, which limits its scope.
What Is Influence Filtering?
Influence Filtering prioritizes content from sources with high influence scores. Platforms like HootSuite use influence scores like Klout to filter tweets.
- Advantages: It offers flexibility and helps users stay informed;
- Drawbacks: The metric for influence is often unreliable and is currently only applicable for real-time feeds like Twitter.
The Blend of Algorithms and Social Graph in Social Search
Social search offers a combo of algorithmic and social graph filtering to find relevant results.
- Advantages: It provides highly relevant results, combining the goal-oriented approach of search with the spontaneity of social aspects;
- Drawbacks: It requires searching and has limited utility for fresh, real-time news.
Harnessing the Potential of Location Filtering
Location filtering leverages your geographical information to provide relevant results— a practice becoming increasingly popular with the rise of the mobile web.
- Advantages: It provides highly relevant results with an impact on your real life;
- Drawbacks: It raises privacy concerns and is currently limited in scope.
No single approach stands out as the best. Relevance is subjective, varying from individual to individual, and situation to situation.
Factors that Determine Success in the Relevance Filtering Space
The success of a platform or service majorly depends on the support for multiple approaches, flexibility in filtering degrees, number of mobile platforms supported, and what users can do with the information presented.
Master the art of Searching Twitter Lists Efficiently, and discover how it complements the ByRelevanceFilter approach in our SEO Strategy guide.
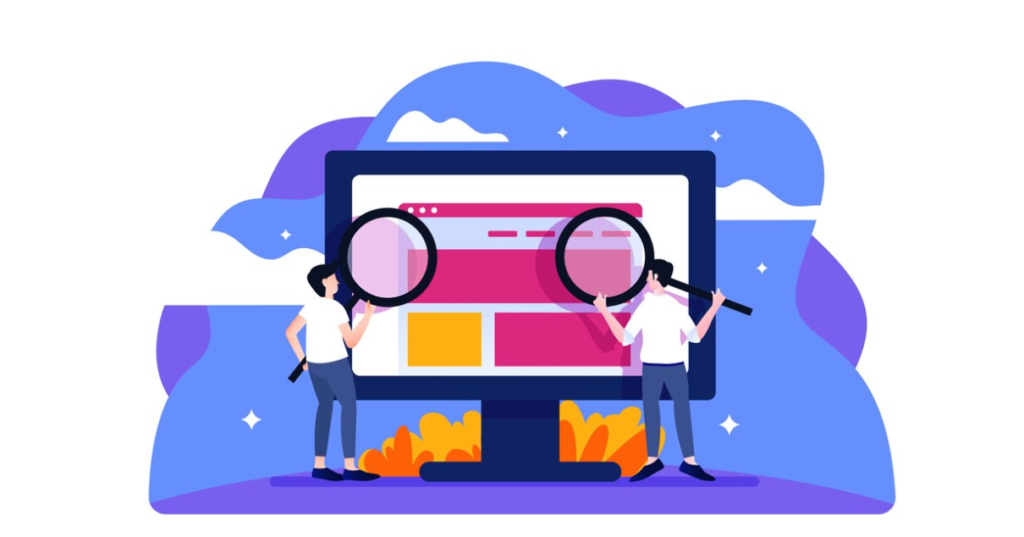
Increasing Importance of Personalized Filtering
Personalized filtering enhances user experience by tailoring content to individuals’ specific interests and consumption habits. It is becoming more prevalent with technological advancements and growing importance of user-centric practices in content delivery.
The future of relevance filtering is promising, with technological advancements enabling more intricate and refined filtering mechanisms. The possibilities are endless, from AI-powered filters to adaptive systems that learn from users’ behavior, the scope for development is enormous.
Conclusion
Achieving relevance in content delivery is a multifaceted challenge. Various filtering approaches cater to different aspects of it, each with its advantages and drawbacks. Personalized filtering and future developments promise enhanced user experiences, but it’s important to keep relevance as the primary focus. The journey to mastering relevance in filtering is ongoing, as user needs and technologies evolve.
It’s crucial to acknowledge that relevance is a fluid concept, varying from person to person and situation to situation. Various filtering methodologies serve as tools to present users with information that suits their interests and needs. Recognizing that no single approach is universally perfect, the key to successful information filtering lies in the balanced application of these diverse methods. Moreover, the integration of these strategies with emerging technologies and users’ evolving preferences should be the cornerstone of future developments in this sphere. As we explore further, these advancements promise to redefine the landscape of content relevance and user experience.